Building AI products: 5 lessons from our founders' workshop
Jasper Masemann, investment partner, and Lutz Finger, venture partner at Cherry Ventures recently held two exclusive workshops for our portfolio companies, one in London and one at the popular startup accelerator Station F in Paris. The workshop gathered over 20 founders to discuss building artificial intelligence (AI) products, and its typical challenges. Here are the lessons learned.
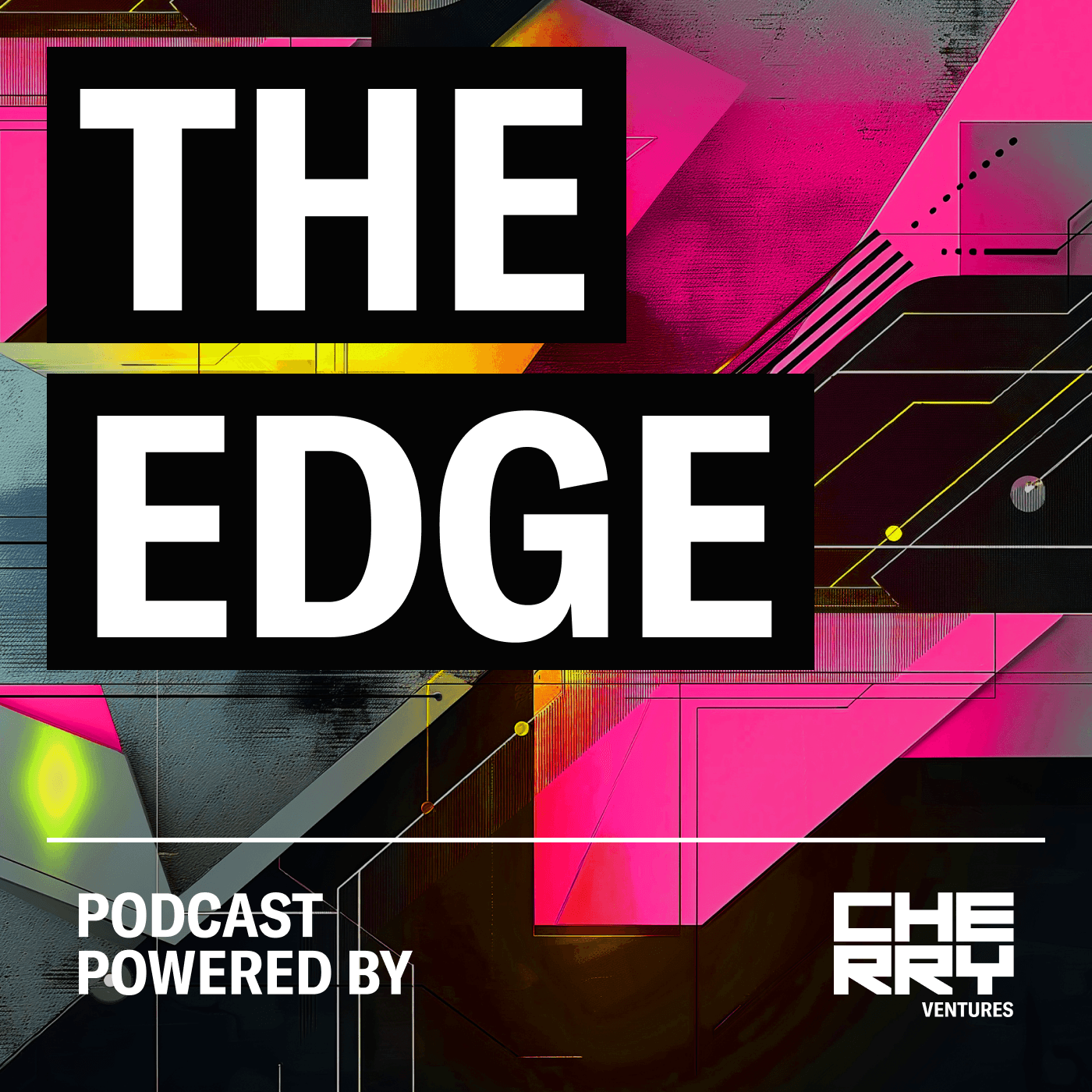
1. Navigate the trial and error for success
Unlike conventional software, AI development involves testing and refining models to find the right fit. For example, a startup aiming to develop an AI-powered customer service chatbot must explore various machine learning models to determine which best understands and responds to user inquiries. This exploration phase is crucial as it requires the team to decide whether the chosen AI technology can effectively solve the problem at hand.
A common pitfall is integrating AI for the sake of novelty rather than necessity. If you can’t identify AI's value proposition, it only becomes an unnecessary complexity. To pinpoint where and why AI adds value to your product, try to understand how AI can enhance user experience, improve efficiency, or provide unique features that traditional methods cannot offer. For example, adding a generative AI feature to a digital weight scale may not add value, but using AI to offer personalized dietary suggestions can significantly enhance the product's core function of weight tracking.
2. Focus on Minimum Viable Quality (MVQ)
Instead of solely focusing on a Minimum Viable Product (MVP), AI-driven projects should prioritize Minimum Viable Quality (MVQ). Consider a company developing an AI system for predictive maintenance in industrial machinery. Here, the accuracy of predictions is crucial, as false positives or negatives can lead to costly downtime or repairs. Having a clear idea of the MVQ ensures that you can confidently deliver a product that meets essential quality standards, even if it's imperfect, as it still addresses critical customer needs.
3. Manage data quality and bias
The effectiveness of an AI model is highly reliant on the quality of its training data. Bias in data is an inevitable challenge since all data is inherently influenced by the conditions and perspectives under which it was collected. For example, if a startup's dataset predominantly reflects a certain demographic, AI may produce biased outputs that fail to generalize across other groups. This is an issue particularly noticeable in social media monitoring or customer service applications.
To mitigate bias, companies can balance datasets to ensure diverse representation or apply statistical methods to correct biases. However, bias can be deliberately maintained if it serves the product's goal. For example, in predictive maintenance for infrastructure, selecting the most relevant and high-quality data, even if it's biased towards the best-case scenarios, can lead to more accurate predictions.
Additionally, while having more data can improve AI models, the focus should be on the quality of the data. For example, in automated accounting systems, a smaller, well-curated dataset is more valuable than a large volume of low-quality data. Startups must, therefore, focus on acquiring not just more data, but the right data.
4. Consider scalability and adaptability right from the start
Scalability and adaptability are crucial for the success of AI products. For instance, while a legal tech startup using AI to analyze contracts might face high initial computational costs, these expenses can decrease as the system processes more data, allowing for economical scaling. Efficient scalability can thus transform a high-cost service into a profitable one. Similarly, adaptability is essential, as seen in healthcare AI systems designed to diagnose specific cancers. These systems must be flexible enough to integrate new medical data and treatment options, ensuring they continue to provide value as the field evolves. By steadily improving and expanding their capabilities, adaptable AI systems not only meet changing user needs but also offer a long-term competitive edge.
5. Don’t be afraid to be the first mover
Companies that successfully integrate AI into their products early on can capture significant market share before competitors. They set industry standards and expectations, which can be difficult for later entrants to match. For instance, early adopters of AI in customer service, like advanced chatbots or predictive maintenance systems, can attract and retain customers with superior solutions.
First movers also benefit from a steep learning curve, refining their AI systems through continuous experimentation and optimization. This allows them to enhance data quality and user interfaces, as seen in healthcare diagnostics, where iteratively improved AI models provide increasingly accurate results, building trust among users.
Moreover, first movers can amass large datasets over time, which are critical for training and improving AI models. This data advantage creates a significant barrier to entry for competitors, as newer entrants may not have access to comparable volumes or quality of data. Additionally, long-term data collection allows these companies to detect trends and fine-tune their AI models, improving decision-making capabilities and product offerings.
Building successful AI-powered products requires a strategic approach that emphasizes clear value creation, quality management, and economic scalability. While being the first mover in AI comes with risks, such as the challenges of uncharted territory and potentially high initial costs, the benefits of establishing market leadership, accumulating data, influencing industry standards, and rapidly iterating on products can far outweigh these challenges. For those willing to take the plunge, the ability to iterate and enhance AI models over time can cement a startup's position as an industry leader.
You can explore these insights and more by tuning into The Edge, our very own podcast breaking down the latest AI developments. We publish a new episode every Thursday, stay tuned!