AI Products: Why ‘Good Enough’ Beats Perfect
While tech giants chase perfection, focused AI startups are winning market share by being "good enough." Ferdinand Terme of Lasqo AI reveals how vertical AI companies succeed by solving specific problems exceptionally well, challenging conventional wisdom about quality and scale in AI. A masterclass in finding product-market fit in today's AI landscape.
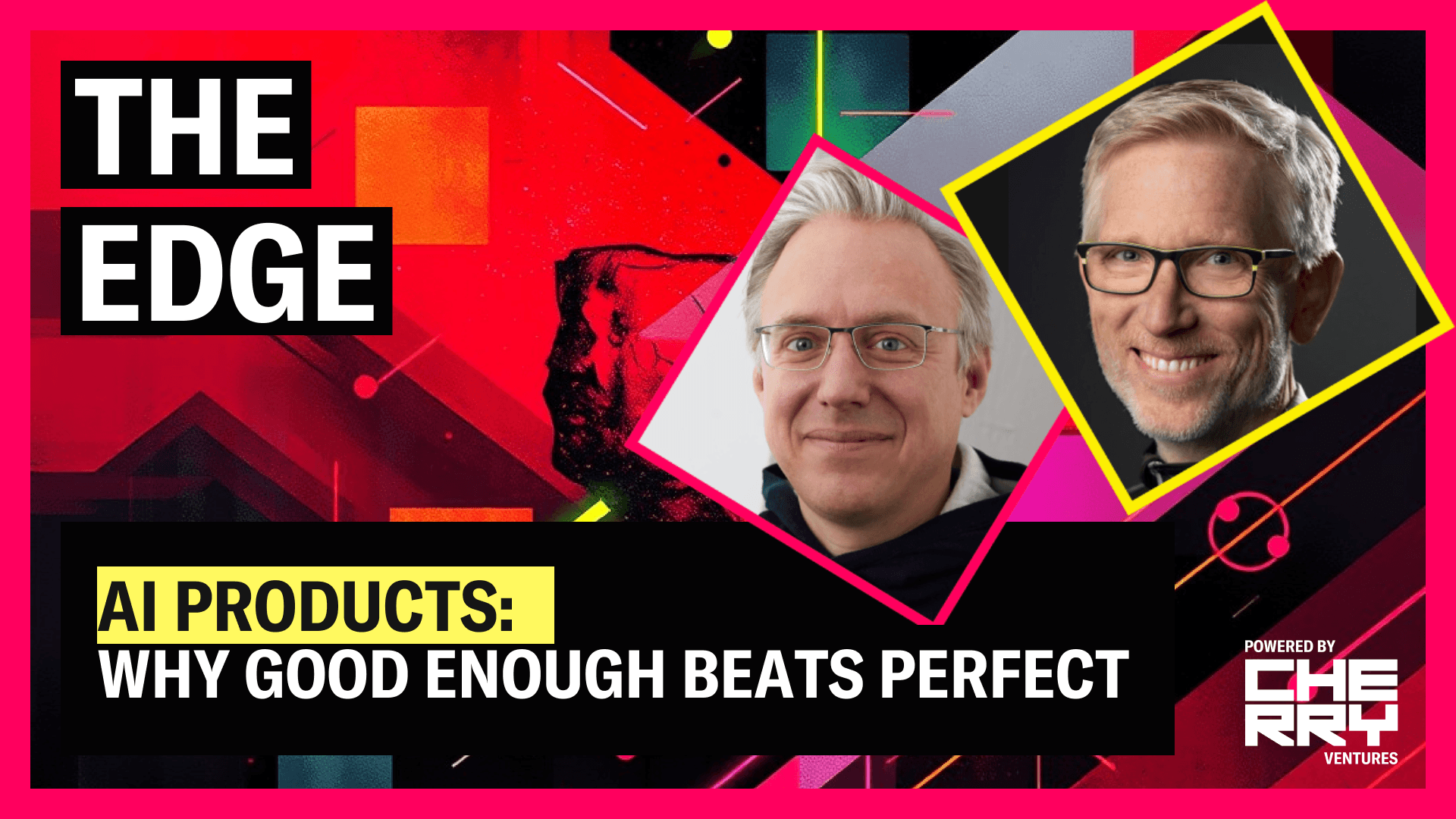
While tech giants struggle with maintaining perception of perfection, a new wave of focused AI companies is rapidly capturing market share. This isn't despite their limitations, but because of them. The story of AI product-market fit is being rewritten by companies that understand: in the messy real world, 'good enough' with the right focus beats theoretical perfection.
The conventional wisdom suggested that AI would follow a familiar pattern: large tech companies would leverage their scale and resources to dominate the application layer. Meta's celebrity chatbots would revolutionise social interaction. Adobe would seamlessly integrate AI into every creative workflow. Anthropic's Claude would redefine knowledge work.
Reality has played out differently. While these companies grapple with maintaining their high standards across broad horizons, a new cohort of highly vertical AI startups is finding success by doing one thing exceptionally well.
We brought Ferdinand Terme from Lasqo AI onto The Edge Podcast, to uncover how, sometimes, being 'good enough' with the right focus beats striving for perfection.
The new wave of highly vertical startups are challenging conventional wisdom about quality and scale in AI.
The Age of Supercreation meets Reality
At Cherry Ventures, our AI thesis posits that we're entering an age of supercreation, where AI supports and enhances human creativity without replacing the unique contributions of artists/designers.
This is where companies like Lasqo are finding their sweet spot. Instead of trying to replace designers, they're augmenting their capabilities and democratising access to design resources.
"We bring today an additional capacity to a company. They don't have a brand design model, they have a very limited bandwidth, and so we enable them to express their brand like they couldn't never have imagined."
For small and medium-sized businesses, maintaining consistent, high-quality brand presence has traditionally been out of reach. AI tools are changing this by making professional-grade design capabilities accessible to marketing teams without extensive design resources.
This approach focuses on augmentation rather than replacement. Rather than trying to replicate human creativity entirely, effective tools focus on removing friction from specific parts of creative processes. A pattern seen in many successful AI implementations.But this focus on specific friction points raises an eternal venture capital question: "Isn't this just a feature?"
The Startup Advantage
While it's easier than ever to create an AI feature, it's harder than ever to build an AI product.
What separates a feature from a product in AI is depth of solution. While Lasqo, on the surface, might seem like "just" an AI image generation feature, dig deeper, and you'll find:
- Complete Workflow Integration: Rather than a standalone capability, it's embedded in the marketing team's daily operations through Slack
- Specialised Training: Custom LoRA models trained on specific brand guidelines
- Human-in-the-Loop Quality Control: A pragmatic approach to ensuring consistent quality
- Feedback Loop Integration: Direct customer interaction enabling rapid iteration
"The cost of the human in the loop is not that high for us because we have a recurring product and we sell in B2B," Ferdinand explains. This integrated approach is what transforms a feature into a product. Features solve tasks, products solve business models.
While Adobe or Canva could theoretically allocate massive resources to replicate these focused solutions, they face a fundamental paradox: the broader your product becomes, the harder it is to go deep in any one direction. The incumbent's dilemma.
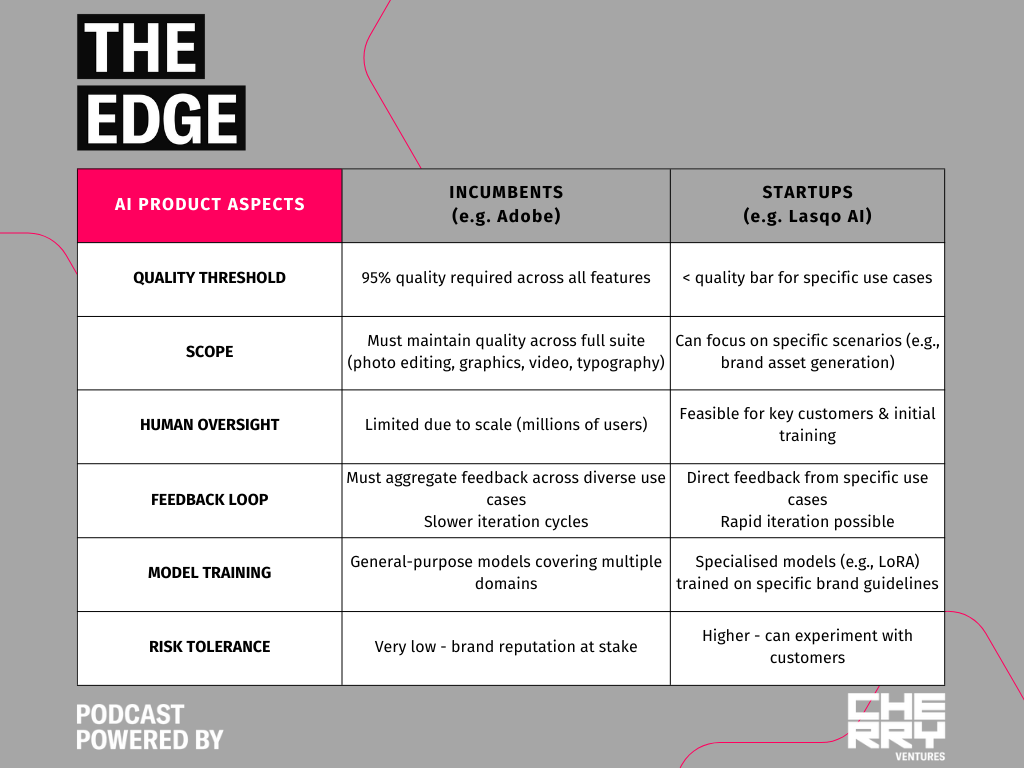
"If tomorrow, Adobe puts $100 million on the table to do exactly what we're doing, I think they will figure it out at some point," Ferdinand acknowledges. But this misses the point.Large companies like Adobe have spent decades building their reputations on predictable, high-quality outputs. Their existing quality control processes are designed for deterministic features, not probabilistic AI outputs. The challenge therefore isn't technical—it's organisational.
Instead, by moving fast and focusing on a single segment with a limited but refined product, startups can:
- Build deeper rather than broader
- Maintain tight feedback loops with specific customer types
- Optimise the entire experience for targeted use cases
- Take more risks in their approach to problem-solving
This focus allows startups to turn what might be just another feature for an incumbent into a full product by going deeper, not wider.When PhotoRoom launched its aim was to be the best at removing backgrounds from photos. Today, it's a $100M ARR business, and a legitimate Photoshop competitor. This pattern will likely repeat across the AI landscape as focused products find their specific sweet spots in the market.
History suggests this is fertile ground for venture returns. During the cloud and mobile transitions, ~20 application layer companies achieved $1Bn+ in revenue.
The Interface Leap and New Tooling
This transformation is more than what these products do, but also how users interact with them. This is where Lasqo's approach aligns with what Cherry calls "The Interface Leap."
For six decades, software interfaces have evolved through accumulation: folders from file cabinets, desktops from actual desks, shopping carts from real stores. Each metaphor built upon the last, creating a comfortable but increasingly antiquated interaction model.
This creates two distinct approaches to AI integration:
The Incumbent Approach: Adding AI features within existing interaction patterns
- AI tools tucked behind traditional menus
- Capabilities fragmented across existing workflows
- Preservation of familiar metaphors
The AI-Native Approach: Building interfaces around AI from first principles
- Natural language as the primary interaction model
- Probabilistic outputs as a core design consideration
- Feedback loops built into every interaction
If you're Canva or Adobe, you cannot say 'OK, let's start from scratch.' Let's just reveal everything with these AI main influences in mind, it's hard for them also to get the right UX.
Free from legacy systems or user expectations, focused startups like Lasqo have an opportunity to define entirely new interaction paradigms for specific use cases.
Ferdinand's team is part of the new tooling wave in AI, "taming" AI for enterprise use.
By transforming static brand guidelines into dynamic AI constraints, building probabilistic quality controls, and embedding capabilities directly in existing workflows. Each interaction refines the system's understanding of business context, turning simple capabilities into deep moats.
As we've seen with previous platform shifts, the companies that win aren't those trying to boil the ocean—they're the ones that start with a focused wedge and expand from a position of strength. In AI, that means being 'good enough' in a specific context may be the best strategy of all.
-
To hear more about building AI products, turning features into companies, and Ferdinand's journey from McKinsey to founding Lasqo, tune into the full episode of The Edge. We dive deeper into topics like human-in-the-loop approaches, scaling AI products, and navigating the incumbent challenge.