AI Deployment: Lost in Translation
Why do most predictive AI models fail to reach production despite proven technical capabilities? Featuring insights from machine learning veteran Eric Siegel on solutions to this critical challenge.
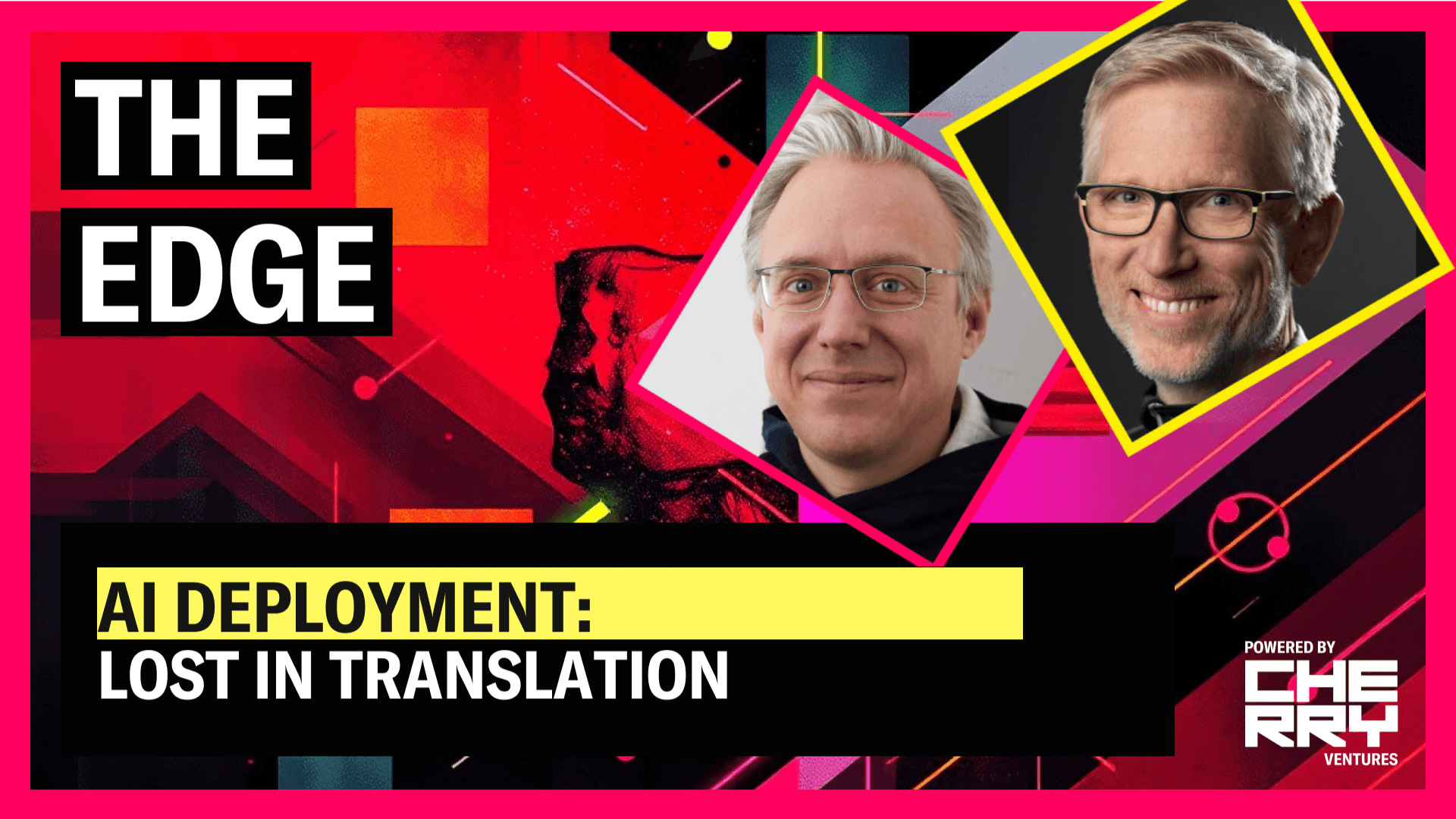
The Value Translation Gap: AI's Deployment Problem
Every day, billions of business decisions hinge on four critical predictions: who will click, buy, lie, or die.
These high-stakes calls aren’t handled by the generative AI apps making headlines or the AGI projects chasing billions. Instead, they rely on Predictive AI quietly transforming enterprise operations.
But there is an irony. While predictive models are proven to deliver millions in value, most never make it to production. There is a fundamental gap between technical insights and business outcomes.
Eric Siegel, a 30-year veteran in machine learning, joins The Edge to unpack why enterprises fail to bridge this divide, leaving transformative potential unrealized.
For investors and founders, this presents both a warning and an opportunity. The companies that succeed in AI will be those that can bridge the gap between technical capability and business value. A challenge of translating theory into usable, daily business solutions.
Why Good Models Die in Development
Despite enterprises investing millions in data science teams and machine learning infrastructure, only 15-20% of predictive models reach production. This mirrors a broader pattern in enterprise AI implementation, where technical metrics impress stakeholders but implementation costs (26%) and disappointing ROI translation (18%) consistently torpedo pilot programs.
The traditional enterprise software playbook - build product, sell seats, deploy technology - fundamentally breaks down with predictive AI.
What’s missing is a framework Eric calls "BizML" that bridges technical performance and business outcomes. At its core are three semi-technical concepts that business stakeholders must grasp:
- What's being predicted
- How well it predicts, and
- What actions those predictions drive.
But even with this framework, models die in development for several critical reasons.
First is the metrics mirage.
"Area under the ROC curve of 0.88. Isn't that awesome?" Eric mimics the typical data scientist's excitement. "All that tells you is the pure predictive performance abstractly, its relative performance comparison to random baseline." While such metrics validate model performance, they fail to answer the crucial question: How much value will this create if deployed?
Second is the workflow-reality gap.
When a model suggests skipping scheduled maintenance on seemingly healthy equipment while increasing inspections on others that appear fine, it challenges decades of operational wisdom. These friction points kill otherwise viable models.
Third is the scale challenge.
Models that shine in controlled pilots often stumble when faced with production data volumes, real-time requirements, and edge cases. Without robust MLOps infrastructure and clear deployment frameworks, promising models remain perpetually stuck in prototype purgatory.
Enter the Translation Layer
Enterprise spending on AI has surged to $13.8 billion in 2024, yet the tools for measuring AI's business impact remain stubbornly technical. This creates a fundamental challenge for predictive AI adoption - how do you value something you can't measure in business terms?
Traditional monitoring tools track technical metrics like precision and inference time, but a new software category is needed to translate these into business KPIs executives value, such as revenue impact, cost savings, and efficiency.
The go-to-market motion for these tools also requires different messaging than traditional ML products. While ML platforms sell to data scientists on technical capabilities, metrics translation tools must speak directly to business stakeholders - CFOs, COOs, and line-of-business leaders who think in terms of risk, return, and operational impact.
For investors, this signals an important shift in the AI tooling landscape. While the last wave focused on model development and training, the next wave will focus on deployment, measurement, and business value realisation.
Product vs. Consulting in AI
The traditional venture thesis says to avoid services businesses - they don't scale. Yet Eric points out that predictive AI inherently requires deep organisational engagement.
The solution lies in building opinionated solutions that encode the patterns of successful deployment into the product itself. "It's not like ML ops or any other kind of technical solution," Eric explains. "It's the organisational procedure, the framework playbook that ensures collaboration in the right way."
This manifests technically in how companies architect their solutions. Instead of building general-purpose models, successful companies are creating domain-specific architectures that embed business logic directly into their ML pipelines.
Taking the example of fraud detection systems. Traditional approaches deliver models that output fraud scores, leaving organizations to figure out implementation. Modern solutions instead embed the entire fraud operations workflow into their architecture. e.g.
- Adjusts detection thresholds during high-volume periods like Black Friday
- Routes cases to investigation teams based on their current capacity and expertise
- Calculates the optimal investigation strategy based on transaction value and risk
- Maintains compliance audit trails and investigation documentation
What was once consulting expertise becomes product functionality. Instead of teaching clients how to operationalize fraud detection, companies build products that encode these best practices into their core architecture. The engineering challenge shifts from improving model accuracy to designing systems that can learn and adapt to each organisation's specific constraints while maintaining consistent deployment patterns.
This approach demands starting with deep vertical expertise. Founders might spend months embedded with fraud operations teams, understanding their workflows, constraints, and decision processes. But unlike traditional consulting, this knowledge gets transformed into product features - automated workflows, intelligent routing systems, dynamic thresholds that adapt to business conditions.
The winners in this space will be companies that successfully productise domain expertise while maintaining the flexibility to adapt to specific business contexts.
Where Smart Money Should Focus
The flood of capital into generative AI and AGI moonshots creates a unique opportunity for investors who understand Eric’s core insight: predictive AI's massive untapped value isn't locked behind technical barriers, but organisational ones.
"We don't have to be irrational," Eric argues, addressing the market's current fixation with AGI. "And even if we do have to be irrational, can we limit that to only being a little irrational?"
For founders and investors, this suggests three clear areas of opportunity:
- Value Translation Tools: The most immediate opportunity lies in building tools that help enterprises understand and capture value from AI. Eric’s own company, Gooder AI, exemplifies this approach by translating technical metrics into business outcomes - a crucial bridge between data science capabilities and P&L impact.
- Vertical Solutions: Rather than chasing broad, horizontal platforms, the winners will likely be companies that deeply understand specific industry workflows and can embed predictive capabilities directly into existing processes. This is particularly true in sectors like healthcare and financial services, where domain expertise matters as much as technical capability.
- Deployment Frameworks: The gap between pilot and production represents a massive opportunity. Companies that can standardize and streamline the deployment process, while accounting for organizational change management, are positioned to capture significant value.
"The antidote to hype is to focus on concrete, credible use cases," Eric reminds us. For investors, this means looking beyond technical capabilities to understanding how effectively companies can bridge the gap between AI potential and business reality. The next wave of successful AI companies won't win by building better models—they'll win by helping enterprises successfully deploy them.
-
To hear more about bridging the AI deployment gap, turning technical metrics into business value, and Eric Siegel's 30-year journey in machine learning, tune into the full episode of The Edge. We explore 'BizML' frameworks, successful model deployment strategies, and navigating enterprise transformation challenges.
These may also interest you
See all ›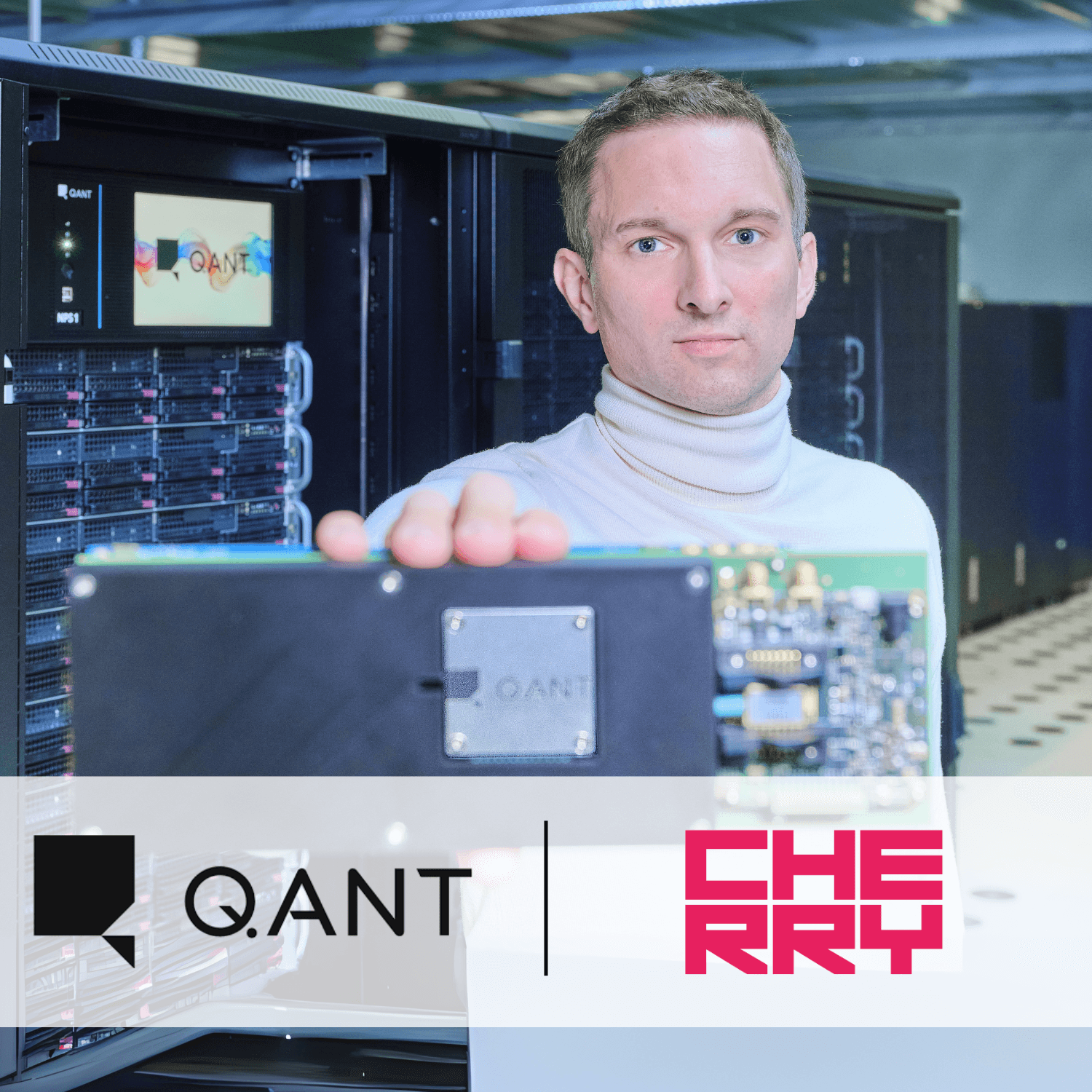
Announcements
Q.ANT’s Photonic Chips Are Rewiring the Future of AIAs AI overheats the planet, Q.ANT is building a cooler alternative - photonic AI accelerators that compute with light, not electricity.
Jul 17, 2025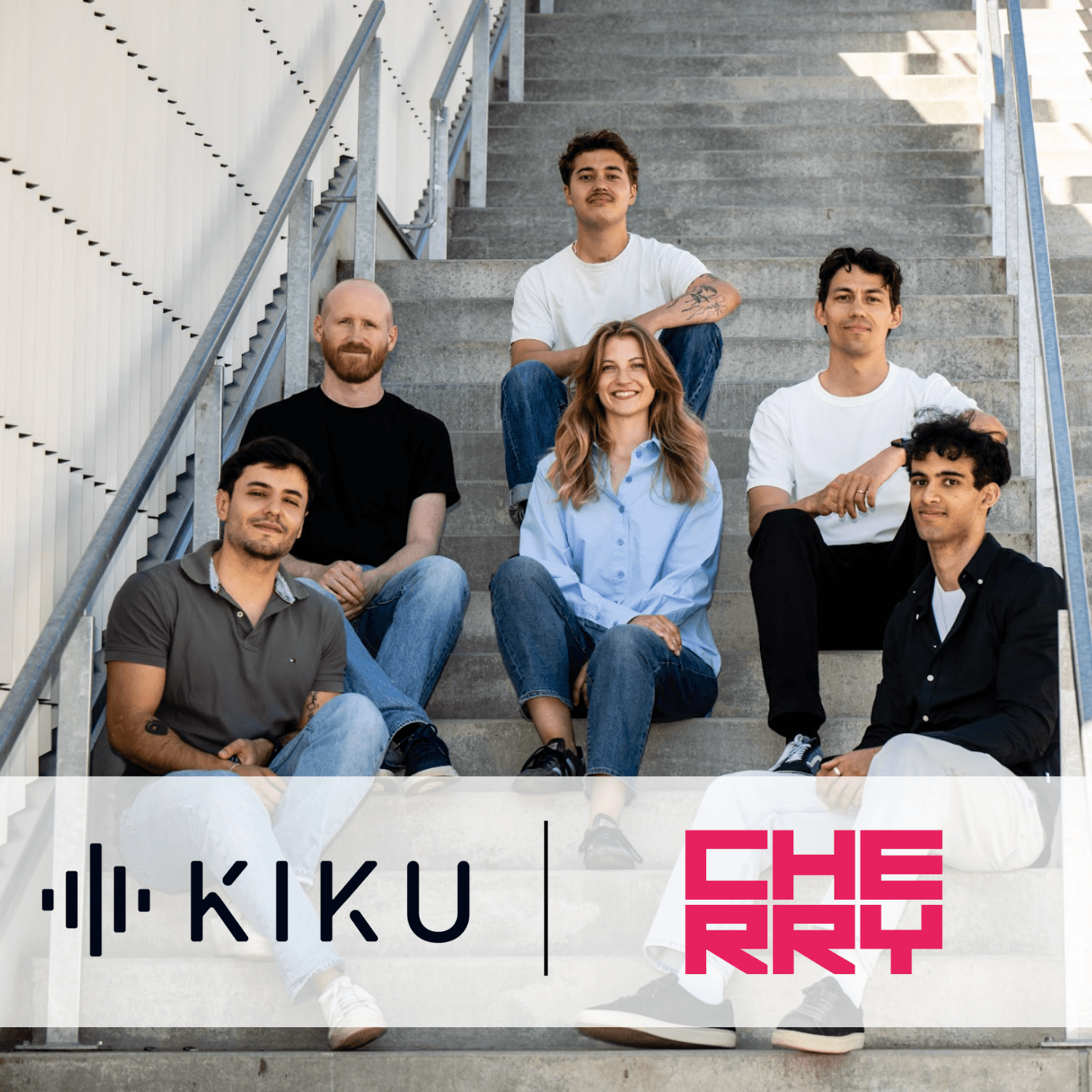
Announcements
Rebuilding Hiring for the People Who Keep the World Running with KIKUCherry Ventures backs Kiku's AI platform that's transforming frontline hiring for 2.7 billion workers. With 93% satisfaction rates, and 75% faster hiring, Kiku is building the infrastructure that makes recruitment work for the people who keep the world running.
Jul 16, 2025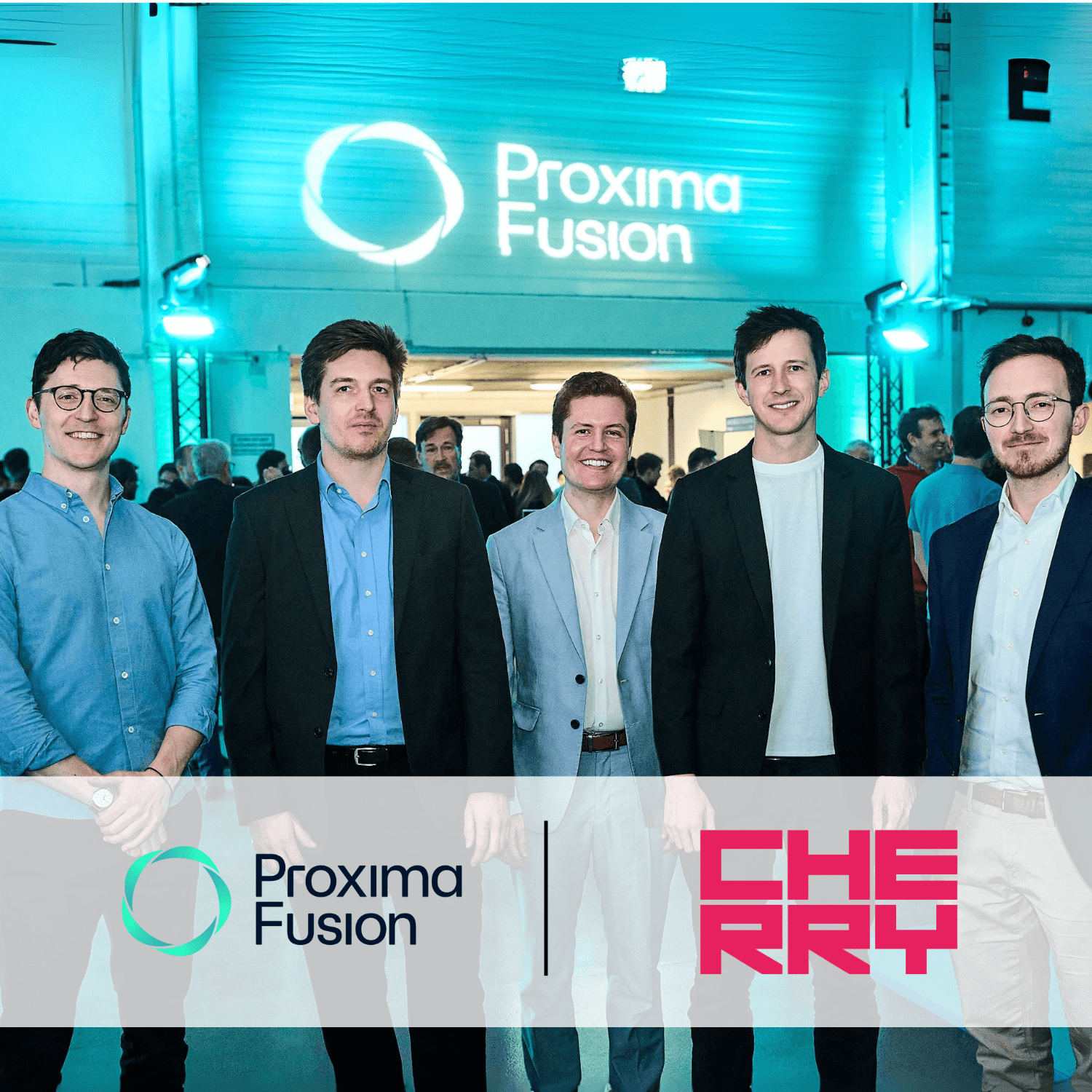
Announcements
Inside Our Investment in Fusion - Why Proxima Could Build Europe's First Trillion-Dollar CompanyIn conversation with Filip Dames, Partner at Cherry Ventures, about investing in Proxima Fusion - Europe's most promising fusion startup.
Jun 11, 2025